Top 7 Challenges Implementing an AI Agent in Customer Support (+ How to Overcome Them)
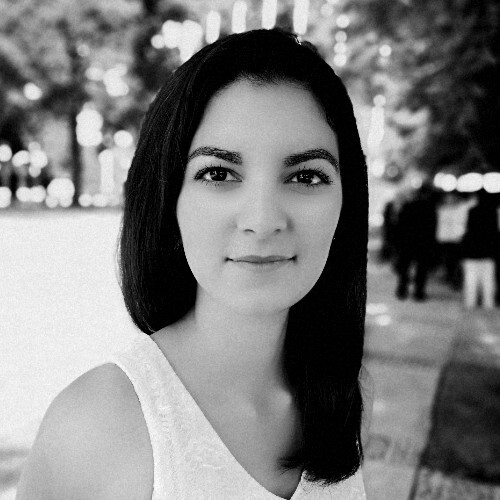

Customer-facing AI agents have been around for a long time.
There’s no shortage of opinions about how or why some customer segments dislike them. But customer sentiment has changed dramatically over the last couple of years, as AI agents are capable of so much more now than ever before.
73% of consumers believe AI can positively impact the customer experience.
The biggest challenge is implementing an AI agent in a way that enables that touch of personalization and care you typically expect from human agents.
That’s the challenge facing many customer support teams today. The good news is that you can approach the implementation in a systematic way and achieve the results you’re looking for.
Here’s how.
The benefits of implementing an AI agent
AI agents come with a ton of impactful benefits.
They offer:
- Increased efficiency. They can handle repetitive and routine tasks, freeing up human agents to focus on more complex and value-added activities–leading to a more productive and efficient support team.
- Higher customer satisfaction. They provide round-the-clock support, reducing response times and ensuring that people can get instant help no matter the time of day. Their responses can also be more consistent. They’ll never have a bad day, get frustrated, or notice that they’re answering the same question for the hundredth time that day.
- Scalability. They can handle an almost infinite number of customer queries without a proportional cost increase, which is especially valuable for those of us dealing with peak seasons or businesses experiencing rapid growth.
- Multilingual support. They can understand and respond in multiple languages, making it easier to support a diverse, global customer base and breaking down language barriers.
- Development opportunities for support agents. Human agents can focus on developing their skills in more complex areas, like revenue generation, technical investigations, or customer relationship management.
And that’s just the tip of the iceberg.
Many companies are seeing massive results from implementing an AI solution because they’ve mastered the art of effective implementation.
Top seven challenges of implementing an AI agent
Setting up a customer-facing AI agent has four broad stages.
- In the planning stage, you decide which tool you want to use and why. It also includes the initial communication to your team.
- The initial rollout phase involves choosing a handful of cases to automate and experimenting with them. At this point, the AI agent interacts with customers but only with a select group.
- Then comes the scaling phase. Once the early kinks are ironed out, scaling and expanding the number of cases that can be automated is the goal.
- Finally, there is the ongoing maintenance stage. When you’re no longer actively scaling, the main focus is on continuous maintenance and monitoring to keep the AI agent updated.
There are some unique challenges at every phase, but there’s also a crossover between these, where you’ll experience the same challenge at multiple stages.
In those cases, the specific solutions might differ depending on where you are in the process–keep that in mind while you’re trying these out.
Overcoming resistance to change
Have you heard of the idiom, “change doesn’t happen overnight”?
AI is a rapidly evolving technology that can be fraught with fear. Your team might be worried about how it will impact them and change their roles. They might also be concerned about how it will impact customers, especially if they’ve had negative experiences with chatbots that haven’t been implemented well.
And these are valid concerns.
You’ll likely face some pushback when thinking about implementing an AI solution. The only way out is through–you’ll have to address every concern wholly and individually. As with any self-service solution, your ultimate goal is to get so much buy-in that your team is the biggest proponent of it.
In practice, that means:
- Involving CS team members in the implementation process. Ask them for their insights about what to automate first and why. Encourage them to identify the repetitive issues that feel monotonous for them.
- Offering training and support to ease the transition. An AI agent has to be deeply embedded in your team. Ideally, everyone should understand how it works and can give feedback or train it directly. At Siena, we offer a comprehensive certification program that anyone can sign up to develop AI skills and acquire the knowledge they need.
- Being very specific and open about your goals. What challenges is your team facing today that you hope AI will help with? How do you think it will impact them? What’s the long-term plan you’re working towards?
There might be resistance to change at every stage of implementation.
Even at ongoing maintenance, there might be an expectation that the AI agent will work autonomously with no instructions or monitoring. That isn’t a realistic outcome to aim for because your product or service will also evolve and change over time. Keep addressing this feedback as and when it comes up.
Selecting the right AI tool
There are an abundance of choices for AI tools right now, and it’s easy to become overwhelmed by the sheer number of options.
- Some tools are designed exclusively for chat, making them ideal for businesses primarily handling live chat.
- Others rely entirely on your knowledge base, providing responses based on pre-existing information.
- Legacy providers might offer a combination of generative AI and rule-based chatbots, which can be great but also have a steep learning curve.
- Your help desk likely offers an add-on with some AI features.
The best way to avoid analysis paralysis is to:
- Set clear criteria for yourself from the beginning
- Prioritize the features you’re looking for based on your business needs.
- Choose the best option to pilot and test it.
This should be a process that takes, at most, a couple of weeks.
Ensuring existing help center content is optimized for AI
Legacy help content often follows a different style unsuited for AI processing.
Think of long tutorials or detailed feature description pages that are lengthy and complex, written for human readers. AI systems require concise, clear, and structured information–and that style is often pretty helpful for customers referring to those articles too.
Updating your help center while implementing an AI agent can be a massive project, but it’s worth the time. It will make it much easier to scale.
- When you’re in the initial rollout stage, conduct an audit of your help center content. It’s a good idea to break up lengthier articles that cover many potential issues into one article per issue.
- Combine article updates with training the AI agent at the ongoing maintenance stage. If you notice that it often references the wrong article, change how that article is written so it’s more precise and clear.
Mastering the learning curve of generative AI
Many CS teams are well-versed in using rule-based chatbots, which follow a predetermined set of rules to provide consistent, but often limited, responses to customer queries.
The transition to generative AI requires a significant mindset shift in training.
- An AI agent built on generative AI will always generate a unique response for that customer. That creates the touch of personalization mentioned earlier, but it also makes it difficult to ensure 100% consistency in the responses.
- Generative AI thrives on context, so it can tailor responses based on that customer and their needs. That means providing additional background information while being very clear about what can be communicated to customers and what can’t in the instructions.
- It can handle ambiguous queries where the question might be vague. But it needs a larger dataset, so it’s always better to provide more information rather than less.
Understanding these nuances in training a generative AI model takes time and experimentation. It can be frustrating at the beginning of the process when you don’t understand why the model behaves in a certain way.
The solution is to:
- Plan for training to take time. Knowing there will be a learning curve means your expectations will be much more realistic.
- Use content from the provider you’re working with. Siena offers a whole certification program specifically to help with this.
- Consider working with an external consultant. If you’re short on time and working towards a deadline, external consultants can help you get started quickly.
Dedicating sufficient resources to scaling it
The initial rollout phase of an AI agent is often the most fun.
There’s usually a lot of momentum. You can quickly automate the easiest and most tedious questions. Focusing on quick wins also means you’ll see quick results.
Getting to that first 20-30% automation rate can happen within a few weeks.
Starting to scale it from there and figuring out great processes to maintain it will require consistent, dedicated resources from your team. The higher the quality of responses you want and the more complex your product, the more dedicated time you’ll need to see those results.
You should:
- Fold it into your OKRs. The only way to make continuous progress is to set clear goals around AI implementation and actively work towards them.
- Assign an owner with dedicated time for it. Ensure someone on your team is responsible for the AI solution and has the time to train it. It’s usually a good idea to select someone quite senior who understands the ins and outs of your product or service very well.
- Potentially incentivize them to reach milestones. Spending time training an AI solution has a massive ROI for your business compared to training an agent for the same time or answering the same questions yourself, because it’s so much more scalable. If bonuses are part of your compensation philosophy, incentivizing reaching certain automation or resolution rates can be hugely beneficial.
Maintaining a human touch in interactions
Maintaining a human touch works by blending the AI with your team.
It might sound counterintuitive but 70% of organizations believe generative AI can help humanize digital interactions.
Not all interactions can or should be handed off to your AI agent. Look at each interaction individually and consciously choose the steps that can be automated.
If the AI agent can handle the issue from end to end and fully resolve it, that’s great. That means customers can expect an instant resolution. Sometimes, it might be better to use it as an assistant that helps you collect the necessary information upfront, or it might be best to provide a personalized acknowledgement and no other information.
The best way to tackle this one is to:
- Implement effective escalation prompts that seamlessly hand conversations to human support when necessary.
- Use an empathic AI model trained to provide personalized responses.
- Define a voice and tone that aligns with your brand’s identity. Consistent communication can massively impact how people perceive the AI agent.
This is something you’ll have to fine-tune and constantly improve over time.
Measuring ROI and performance
It’s tempting to aim for perfection when implementing an AI solution.
Generally, a good benchmark is achieving a balance where the AI can handle routine queries with high precision while seamlessly escalating issues it can’t solve to human agents.
Remember that a 100% accuracy rate isn’t possible (and is difficult to achieve with human support agents!). Above 90% accuracy already ensures that customers get quick solutions without compromising quality.
That’s why figuring out ways to demonstrate tangible business outcomes is important at all stages of implementation. Initially, improving CX metrics can be the primary goal. Over time, you can also track how it impacts customer loyalty, retention, and revenue generation.
To measure ROI and the AI agent’s performance:
- Define clear KPIs. You can structure your goals around KPIs like response time, resolution rate, or escalation rate.
- Compare qualitative metrics. You can compare the AI agent's performance regarding CSAT or CES against your CS team. Sometimes it might be better to compare IQS since external factors will impact that less. Siena calculates a CXP score, which combines multiple factors like CSAT, sentiment, response accuracy, issue resolution, empathy, and ease of handoffs where necessary
- Measure the cost per ticket. Analyze and compare the cost per ticket for interactions resolved by human agents against those handled by the AI.
An AI agent that transforms your customer experience
Embedding an AI agent in your customer support operations can revolutionize your interactions with your customers.
Tackling these challenges head-on will ensure you hit the right balance of automation and personalization. When implemented right, AI can deliver empathetic, context-aware responses that make customers feel valued and understood.
Want to take your customer experience to the next level?
Siena offers an empathic AI agent designed to understand and respond to customer emotions, providing personalized, human-like interactions. With Siena, you can streamline your support operations and create meaningful, positive connections with your customers.
Book a free demo with us today!